06 Life Expectancy 80 60 50 09 In certain settings, when we model Y as a function of X, the relationship does not appear to be very linear. Sometimes, log transformations are applied to the variables to improve the linear fit of the relationship. This is especially common in settings in which one of the variables has extreme outliers. The first figure below shows the results of a linear regression between life expectancy and average income (1000s). The second shows the results of a linear regression between life expectancy and log average income. Which regression appears to better fit the data (i.e. in which figure does it appear that the observations more closely overlap with the linear regression line)? 06 90 Regression of Life Expectancy on Average Income Life Expectancy 70 08 50 50 60 60 °° 50 0000 ° ° 00 Average Income (1000s) o Life Expectancy Fitted values ° 100 Regression of Life Expectancy on Log Average Income ° ° 8 10 Log Average Income o Life Expectancy. Fitted values ° T 12
06 Life Expectancy 80 60 50 09 In certain settings, when we model Y as a function of X, the relationship does not appear to be very linear. Sometimes, log transformations are applied to the variables to improve the linear fit of the relationship. This is especially common in settings in which one of the variables has extreme outliers. The first figure below shows the results of a linear regression between life expectancy and average income (1000s). The second shows the results of a linear regression between life expectancy and log average income. Which regression appears to better fit the data (i.e. in which figure does it appear that the observations more closely overlap with the linear regression line)? 06 90 Regression of Life Expectancy on Average Income Life Expectancy 70 08 50 50 60 60 °° 50 0000 ° ° 00 Average Income (1000s) o Life Expectancy Fitted values ° 100 Regression of Life Expectancy on Log Average Income ° ° 8 10 Log Average Income o Life Expectancy. Fitted values ° T 12
Operations Research : Applications and Algorithms
4th Edition
ISBN:9780534380588
Author:Wayne L. Winston
Publisher:Wayne L. Winston
Chapter24: Forecasting Models
Section: Chapter Questions
Problem 6RP
Related questions
Question

Transcribed Image Text:06
Life Expectancy
80
60
50
09
In certain settings, when we model Y as a function of X, the relationship does not appear to
be very linear.
Sometimes, log transformations are applied to the variables to improve the linear fit of the
relationship. This is especially common in settings in which one of the variables has extreme
outliers.
The first figure below shows the results of a linear regression between life expectancy and
average income (1000s). The second shows the results of a linear regression between life
expectancy and log average income. Which regression appears to better fit the data (i.e. in
which figure does it appear that the observations more closely overlap with the linear
regression line)?
06
90
Regression of Life Expectancy on Average Income
Life Expectancy
70
08
50
50
60
60
°°
50
0000
°
°
00
Average Income (1000s)
o Life Expectancy
Fitted values
°
100
Regression of Life Expectancy on Log Average Income
°
°
8
10
Log Average Income
o Life Expectancy.
Fitted values
°
T
12
Expert Solution

This question has been solved!
Explore an expertly crafted, step-by-step solution for a thorough understanding of key concepts.
This is a popular solution!
Trending now
This is a popular solution!
Step by step
Solved in 2 steps

Recommended textbooks for you
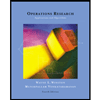
Operations Research : Applications and Algorithms
Computer Science
ISBN:
9780534380588
Author:
Wayne L. Winston
Publisher:
Brooks Cole
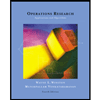
Operations Research : Applications and Algorithms
Computer Science
ISBN:
9780534380588
Author:
Wayne L. Winston
Publisher:
Brooks Cole