Consider the linear regression model y = X3 + u with iid random variables and assume u ~ N(0,0²In). Conditional on X, show that the MLE estimator of 3 coincides with the OLS estimator of 3. Compare the Gauss-Markov Theorem to the Cramer-Rao Theorem in this case. Conditional on X, y ~ N(XB, o² In) and hence f(y|X, 3,0²) = = From here obtain the log-likelihood as n (2π0²)-¹/² exp i=1 (2πо²)-¹/² exp (Yi - X₁3)² 20² (y - XB)' (y - X³) 20² l(y|X, 3,0²) = − (n/2) ln(2ño²) – (y — Xß)' (y – Xß) 20² (2) Since the only term in (2) that depends on ß is the numerator of the second RHS term, maximizing l(y X, B, 0²) w.r.t 3 is equivalent to maximizing - (y - XB)' (y - XB) w.r.t. ß in that both yield the same argmax. At the same time, - (y - XB)' (y - X³) = -u'u n -Σu² i=1 (3) which is the negative of the sum of square residuals criterion function of OLS. Hence maximizing (y|X, B, 0²) in (2) to obtain BML yields the same result as minimizing ₁ u²in (3) to obtain BOLS. The GM and CR Theorems also coincide in this case, since we are applying MLE to a linear model.
Consider the linear regression model y = X3 + u with iid random variables and assume u ~ N(0,0²In). Conditional on X, show that the MLE estimator of 3 coincides with the OLS estimator of 3. Compare the Gauss-Markov Theorem to the Cramer-Rao Theorem in this case. Conditional on X, y ~ N(XB, o² In) and hence f(y|X, 3,0²) = = From here obtain the log-likelihood as n (2π0²)-¹/² exp i=1 (2πо²)-¹/² exp (Yi - X₁3)² 20² (y - XB)' (y - X³) 20² l(y|X, 3,0²) = − (n/2) ln(2ño²) – (y — Xß)' (y – Xß) 20² (2) Since the only term in (2) that depends on ß is the numerator of the second RHS term, maximizing l(y X, B, 0²) w.r.t 3 is equivalent to maximizing - (y - XB)' (y - XB) w.r.t. ß in that both yield the same argmax. At the same time, - (y - XB)' (y - X³) = -u'u n -Σu² i=1 (3) which is the negative of the sum of square residuals criterion function of OLS. Hence maximizing (y|X, B, 0²) in (2) to obtain BML yields the same result as minimizing ₁ u²in (3) to obtain BOLS. The GM and CR Theorems also coincide in this case, since we are applying MLE to a linear model.
Oh no! Our experts couldn't answer your question.
Don't worry! We won't leave you hanging. Plus, we're giving you back one question for the inconvenience.
Submit your question and receive a step-by-step explanation from our experts in as fast as 30 minutes.
You have no more questions left.
Message from our expert:
Our experts are unable to provide you with a solution at this time. Try rewording your question, and make sure to submit one question at a time. We've credited a question to your account.
Your Question:
please only do: if you can teach explain steps of how to solve each part
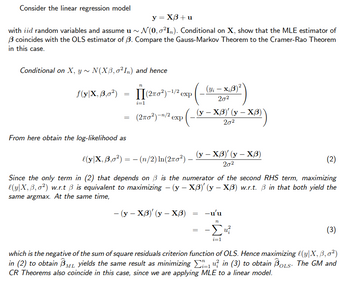
Transcribed Image Text:Consider the linear regression model
y = X3 + u
with iid random variables and assume u ~ N(0,0²In). Conditional on X, show that the MLE estimator of
3 coincides with the OLS estimator of 3. Compare the Gauss-Markov Theorem to the Cramer-Rao Theorem
in this case.
Conditional on X, y ~ N(XB, o² In) and hence
f(y|X, 3,0²)
=
=
From here obtain the log-likelihood as
n
(2π0²)-¹/² exp
i=1
(2πо²)-¹/² exp
(Yi - X₁3)²
20²
(y - XB)' (y - X³)
20²
l(y|X, 3,0²) = − (n/2) ln(2ño²) – (y — Xß)' (y – Xß)
20²
(2)
Since the only term in (2) that depends on ß is the numerator of the second RHS term, maximizing
l(y X, B, 0²) w.r.t 3 is equivalent to maximizing - (y - XB)' (y - XB) w.r.t. ß in that both yield the
same argmax. At the same time,
- (y - XB)' (y - X³)
=
-u'u
n
-Σu²
i=1
(3)
which is the negative of the sum of square residuals criterion function of OLS. Hence maximizing (y|X, B, 0²)
in (2) to obtain BML yields the same result as minimizing ₁ u²in (3) to obtain BOLS. The GM and
CR Theorems also coincide in this case, since we are applying MLE to a linear model.
Recommended textbooks for you
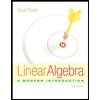
Linear Algebra: A Modern Introduction
Algebra
ISBN:
9781285463247
Author:
David Poole
Publisher:
Cengage Learning
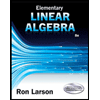
Elementary Linear Algebra (MindTap Course List)
Algebra
ISBN:
9781305658004
Author:
Ron Larson
Publisher:
Cengage Learning
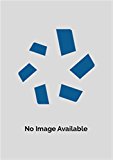
Big Ideas Math A Bridge To Success Algebra 1: Stu…
Algebra
ISBN:
9781680331141
Author:
HOUGHTON MIFFLIN HARCOURT
Publisher:
Houghton Mifflin Harcourt
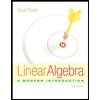
Linear Algebra: A Modern Introduction
Algebra
ISBN:
9781285463247
Author:
David Poole
Publisher:
Cengage Learning
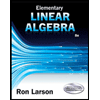
Elementary Linear Algebra (MindTap Course List)
Algebra
ISBN:
9781305658004
Author:
Ron Larson
Publisher:
Cengage Learning
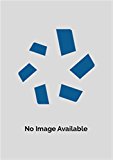
Big Ideas Math A Bridge To Success Algebra 1: Stu…
Algebra
ISBN:
9781680331141
Author:
HOUGHTON MIFFLIN HARCOURT
Publisher:
Houghton Mifflin Harcourt